Data Products 101: What Is a Data Product?
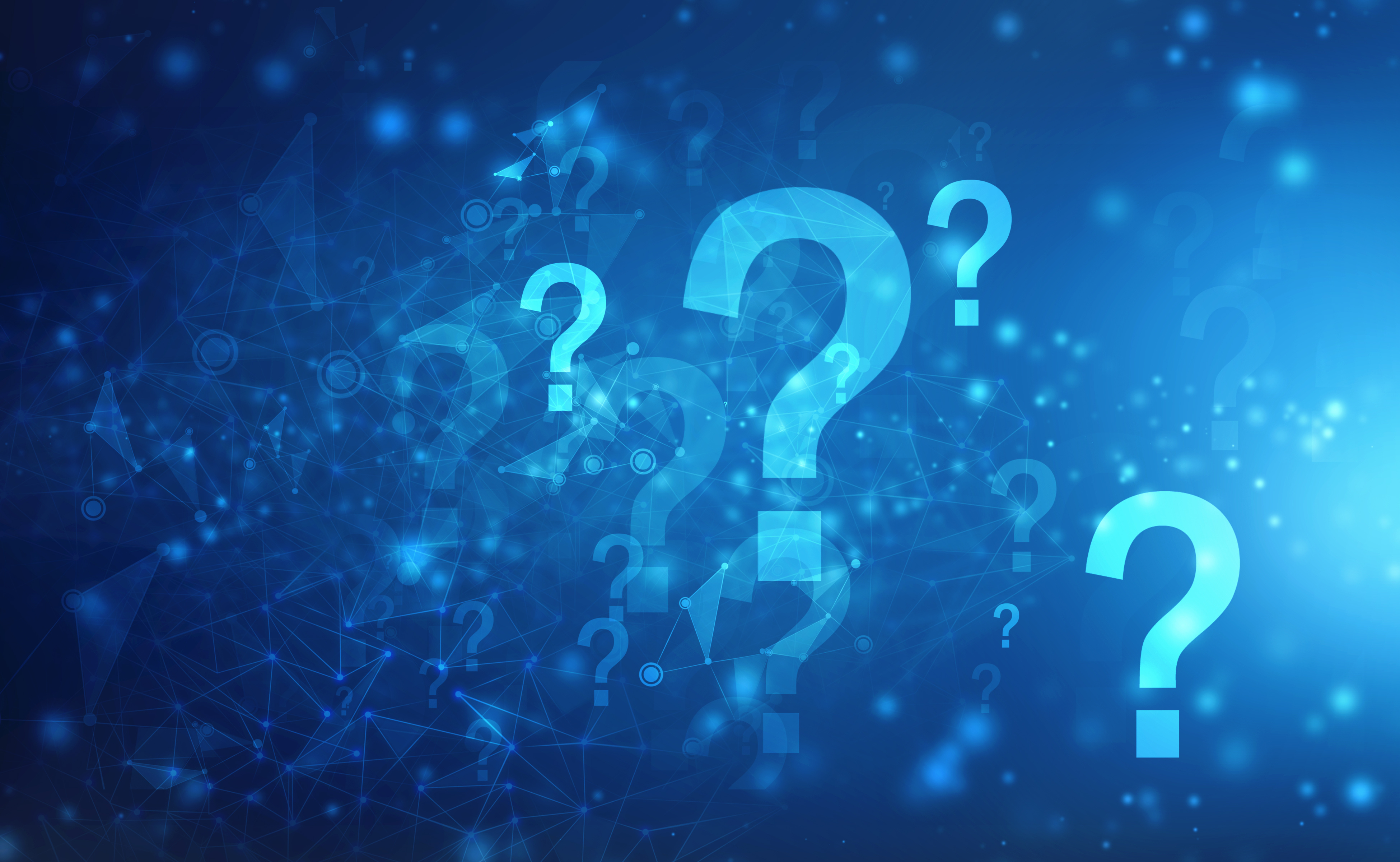
In the past year or two, the term "data product" has become increasingly prominent, with Google searches for what is a data product exploding in popularity since early 2022. The relative recency of the widespread use of "data product" means that it’s still loosely defined and, in a sense, not fully understood. In this comprehensive overview, we’ll define what is a data product, explore the fundamentals, and explain the role they play in driving value and insights for organizations across various industries.
Understanding Data Products
At its core, a data product is a tangible output resulting from the processing, analysis, and interpretation of data. What is a data product, then? It takes raw data—like datasets—and transforms it into valuable insights, predictions, or visualizations. A data product can take many forms, from simple reports and dashboards to complex machine learning models and predictive analytics tools.
All data products rely on data as the primary input, emphasizing the extraction of meaningful patterns, trends, and knowledge to create value. Once defined, these data products are designed for internal use within an organization or for external consumption by customers and stakeholders, providing actionable intelligence to drive decision-making.
The Evolution of Data Products
The concept of data products has evolved significantly in recent years, mirroring the similarly rapid pace of advancements in technology and the increasing importance of data-driven decision-making. In the early days of computing, data was primarily treated as a byproduct of business operations, with limited emphasis on extracting actionable insights. As technology progressed, businesses recognized the untapped potential within their datasets.
The advent of relational databases and business intelligence tools in the 1980s marked a significant milestone in the evolution of data products. Organizations started to recognize the need for structured storage of data and tools that could facilitate analysis. This era laid the foundation for the emergence of reports and dashboards as the first wave of data products, providing users with visual representations of key performance indicators and business metrics.
Since then, we’ve seen a major shift with the rise of big data and advanced analytics. Organizations are grappling with massive amounts of structured and unstructured data—which led to the creation of more sophisticated data products. Machine learning algorithms and predictive analytics became integral components, allowing for real-time insights and more accurate decision support systems. This phase marked the transition from static reports to more dynamic, interactive data products capable of adapting to changing business needs.
Key Components of Data Products
What is a data product, and what makes it so impactful? Data products are complex, taking distributed raw data and turning it into valuable information, knowledge, or actionable insights for users. At a high level, that transformation entails four key steps:
- Data collection and storage: A data product wouldn’t exist if we didn’t have raw data that we wanted to use more effectively, so the foundation of any data product requires the collection and storage of relevant data. This involves identifying sources, ensuring data quality, and establishing robust storage infrastructure.
- Processing and analysis: Before the end user ever sees the outcome, the data product must be able to successfully process and analyze data, often through statistical techniques, machine learning algorithms, and other analytical methods to derive meaningful insights.
- Visualization and communication: Communicating information in a way that’s digestible for users is crucial. Data products often incorporate visualizations, dashboards, and reports to make complex information easily understandable and actionable for end-users.
- Integration: Finally, data products rarely exist on their own; instead, they typically must integrate with existing systems, applications, or workflows to ensure seamless adoption and utilization within an organization. The last step of the data product transformation process must then entail making sure the product can “talk” to its surrounding environment.
Real-World Applications of Data Products
What is a data product in action? In modern, data-driven businesses, there is no shortage of opportunities for data products to be put to use. Rather than being used solely by more technical organizations, data products are versatile and able to deliver value in applications used across diverse industries.
- In e-commerce, recommendation engines, personalized marketing campaigns, and demand forecasting models are examples of data products that can enhance customer experiences and optimize operations.
- In healthcare, data products can exist as predictive analytics for patient outcomes, population health management tools, and diagnostic support systems, contributing to improved decision-making and patient care.
- In the finance sector, fraud detection algorithms, credit scoring models, and portfolio optimization tools are commonly used to aid in risk management and strategic investments.
- And even within manufacturing, data products can be incorporated as predictive maintenance systems, supply chain optimization tools, and quality control algorithms that improve efficiency and avoid downtime.
Considerations to Keep in Mind When Evaluating Data Products
While data products offer immense potential, they come with their set of challenges. Privacy concerns, data security, and ethical considerations are often the biggest obstacles, especially when dealing with sensitive information. Additionally, organizations need to address issues related to data quality, integration complexities, and continually evolving rules and regulations.
The talent gap in data science and analytics also poses a challenge. Building and maintaining effective data products require skilled professionals capable of navigating complex datasets and leveraging advanced analytical techniques. Organizations must invest in talent development and recruitment to ensure they have the expertise needed to derive maximum value from their data assets. Barring this, businesses can turn to designated platforms that make it easy for users of any skill level to create and deliver trusted data products.
The Future of Data Products
Data products have already emerged as indispensable tools in the business world, driving informed decision-making and fostering innovation. As technology continues to advance, the future of data products holds exciting possibilities, helping to democratize data and empower individuals across organizations to better use their data to support them in their day-to-day.
Contact RightData For Your Data Product Needs
RightData is the low-code, generative AI-powered Data Products Platform that powers everyone from the Fortune 500 to small start-ups. Contact our expert team to learn more about how RightData's solutions can transform your data products seamlessly.