Data Observability: ETL Monitoring and Data Validation (Clario)
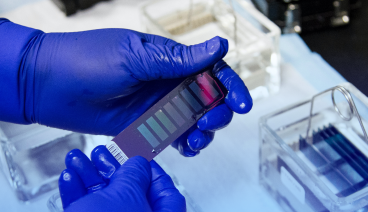
The Need
Clario, a provider of health outcomes research, provides mission-critical, software-enabled clinical research facilities and services, including cardiac safety, electronic clinical outcomes, and suicide risk assessments. Quality data capture and monitoring throughout their ETL process is critical to ensuring load to their Reltio Master Data Management (MDM) solution.
With strict internal Service Level Agreements (SLAs), Clario requires error reporting from various sources at the onset. It is often too late to fix errors quickly when data moves downstream to a Tableau reporting. Lack of trust in the data at the reporting level was causing major issues with clients who depended on Clario for clean and timely data.
The Solution
Clario already had DataTrust (formerly called RDt) installed for classic data quality and validation when the Tech Director recommended extending DataTrust’s use for greater monitoring. DataTrust was further used to monitor the ETL logs and provide process protection and remediation if any of the data failed. DataTrust went beyond monitoring and became more important to the DevOps to DataOps cycle.
In addition, because the data could be designated as either active or inactive, DataTrust could track monitoring against that process metric and provide a profile of validation from upstream sources all the way downstream to where the data repository staged the data for import to Tableau. Clario leveraged the DataTrust software to unify data quality across the enterprise with an automated process.
ETL Monitoring with Source to Target reconciliation
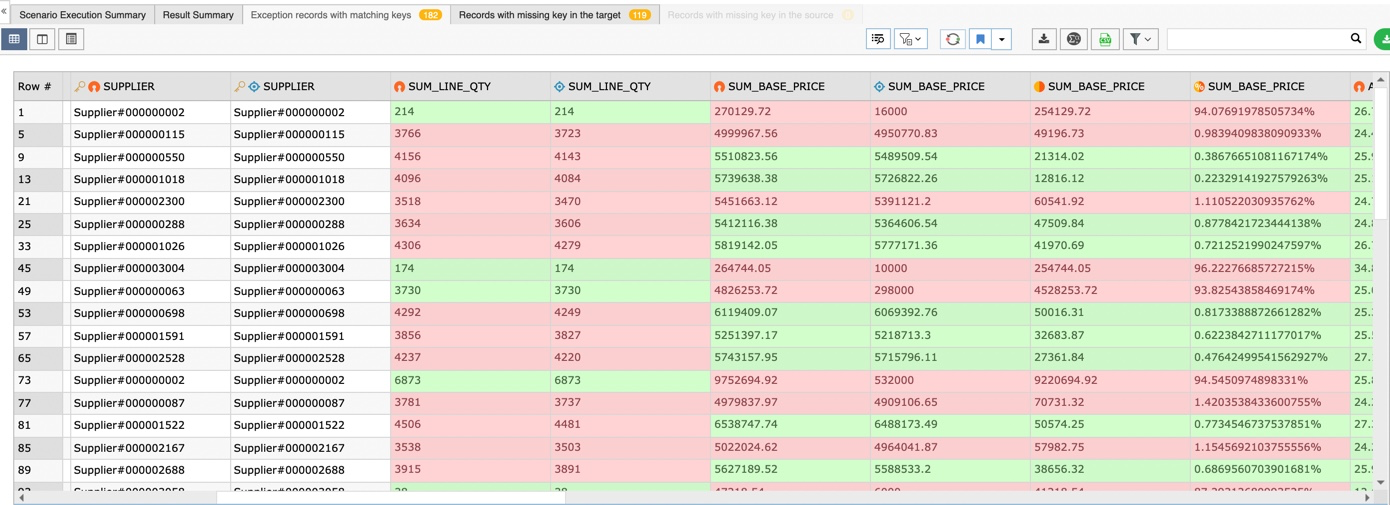
Impact
Robust monitoring of ETL-produced data can be challenging. Ingesting from different sources complicates the workflow since not all sources are alike. Cleansing data is vital to getting better results, but cleansing it quickly is even more critical to timely decision-making processes that affect your business's trajectory. You need reliable data validation and observability to ensure your data is accurate, actionable, and available when you need it.
DataTrust's machine learning capabilities allow you to automatically find and alert on data issues. With help from the software's automation features, you can better allocate resources while ensuring data quality. DataTrust can also automatically generate rules for future data profiling, increasing your time and cost savings.
The further impact is the trust you can build with internal clients with SLAs as you remediate errors within a few hours. When you can quickly check the source of errors and have a metric and alarm before the error hits the final repository, you can save significant amounts of time and produce the quality data your clients expect.
The RightData Edge
Clario provided feedback on DataTrust software and its functionality for both data validation and data monitoring. The edge that RightData provided strengthens the position of the internal data team tasked with the integrity of the data quality. If something goes wrong, the internal data team can fix it easily with the DataTrust software.
Clario explains further:
“There’s a lot of pressure to perform when we are meeting both internal and external data expectations for major pharm clients. We’ve been in business since 1972 and a hallmark of Clario is that clients trust us. Today, we have to demonstrate that they can trust every aspect of data as well… RightData is very much a part of that.”
Learn more about DataTrust
DataTrust is a comprehensive software suite for automated data quality and alerting. It enables continuous, automated data observation, verification, and reconciliation so you can have confidence in your business data's accuracy. From a single code-free, easy-to-use software suite, your internal data professionals can profile data, monitor pipelines, confirm data quality, and detect issues to ensure your data is reliable.
Contact us today to learn how DataTrust works at a massive scale and with minimal effort to ensure your data looks exactly like it should. Our team is available to chat about your needs.
DataTrust Data Quality: A no-code data quality suite that improves data quality, reliability, consistency, and completeness of data. Data quality is a complex journey where metrics and reporting validate their work using powerful features such as:
Database Analyzer: Using Query Builder and Data Profiling, stakeholders analyze the data before using corresponding datasets in the validation and reconciliation scenarios.
Data Reconciliation: Comparing Row Counts. Compares number of rows between source and target dataset pairs and identifies tables for the row count not matching.
Data Validation: Rules-based engine provides an easy interface to create validation scenarios to define validation rules against target data sets and capture exceptions.
Connectors For All Type of Data Sources: Over 150+ connectors for databases, applications, events, flat file data sources, cloud platforms, SAP sources, REST APIs, and social media platforms.
Data Quality: Ongoing discover that requires a quality-oriented culture to improve the data and commit to continuous process improvement.
Database Profiling: Digging deep into the data source to understand the content and the structure.
Data Reconciliation: An automated data reconciliation and the validation process that checks for completeness and accuracy of your data.
Data Health Reporting: Using dashboards against metrics and business rules, a process where the health and accuracy of your data is measured, usually with specific visualization.