Getting Started with Data Products Series: Part Three
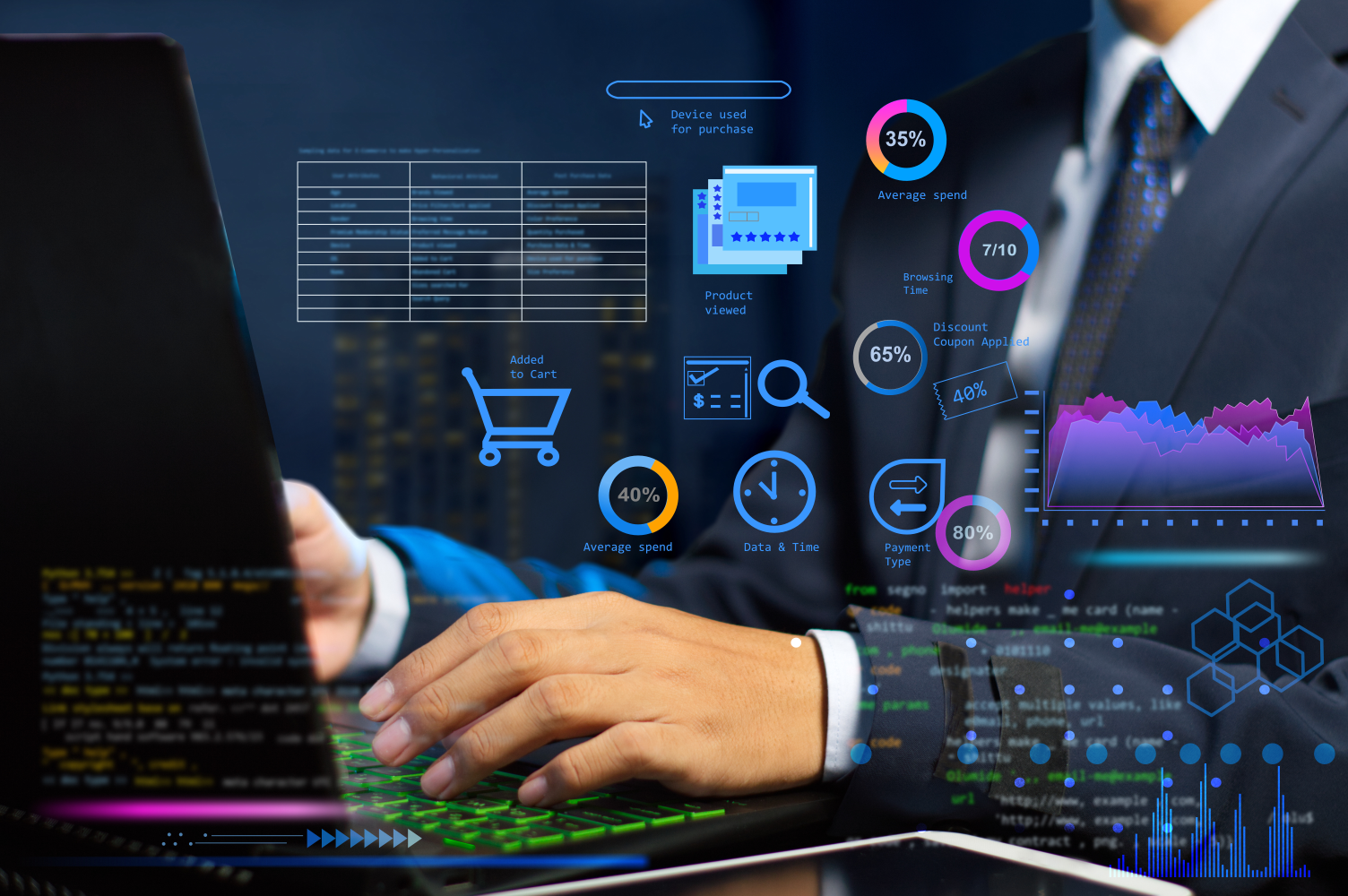
In the first part of this series, we covered the basics of data products. Then, the first two factors you need to consider when building data products. Now, let’s get into the last two factors to keep in mind...
3. Readiness
Data products deliver datasets that are ready for analysis, with high-quality, well-organized, and well-documented data that facilitates decision making and enables engineers to deliver the necessary data to consumers in a timely and efficient manner.
· What kind of data is required to ensure successful use of this data product?
Data products should be created on top of cleansed data. For successful implementation, an organization will need to take its raw or distributed data and bring it together into a dataset that’s ready for consumption with all the data attributes ready for analysis—no enrichment required.
· What does the process of getting my data ready for a data product look like?
Ideally, the data product provider will work with an organization to take the raw data and make it analytics-ready, providing a trust layer on top of it, then data market so you can convert that into data products and make it into a findable market. Ask whether this is part of the provider’s offering or whether your organization will need to handle this on its own.
4. Trust
Data-driven decision-making relies on accurate and reliable data. Data products promote trust by implementing data quality automated software, providing strong data lineage, and establishing data governance policies. This is crucial for data engineers working on data pipelines, as the accuracy of the data they work with directly impacts the success of their projects.
· How is data lineage tracked?
Providing information on data lineage gives users confidence that the data they’re using is correct and auditable. Confirm that the data product’s metadata features fields outlining the data’s original source, specifying how many transformations the data has been through already, whether the data has been audited, who the owner is, and quality metrics, among others.
· What kind of quality metrics will we be able to use?
Every data product needs to have a data quality metrics and historical data quality metrics. Whatever data product is being evaluated, it should feature a rating system for data that includes the number of users who have rated it. Providing this kind of transparency into the quality of the data product itself helps to build trust in data among users. For example, an online shopper is unlikely to purchase a product with zero ratings at all, and they’re also more likely to purchase a product with 2,000 reviews and a 4.5-star rating than they are to purchase a 5-star product with two ratings. Providing this insight into historical quality and performance enables users to feel confident when relying on data.
· How are errors or quality issues within a data product addressed?
If the data is incorrect, a data product needs to have a built-in way to let the data owner know. With a notification system integrated within the platform itself, a data product can help remove barriers to addressing quality issues by making it easier for any user to immediately alert owners of errors or failures so they can be repaired.
Overall, data products can promote trust by providing transparency, consistency, security, strong data governance, and feedback and collaboration mechanisms. By promoting these qualities, users can have greater confidence in the data being analyzed and the insights being derived from it.
Best practices for moving to a data product-centric platform
Moving to a new system that creates actionable data products can feel overwhelming, especially when an organization faces continuing legacy operations with older tools in place. With this anticipated complexity, companies may decide to stick with the status quo—opting for the devil they know over the unknown. However, following some best practices can help make the transition smoother and more successful, resulting in faster adoption rates and a more seamless experience for users.
First, as you begin reviewing possible data products, make sure that all of the necessary stakeholders are involved in the evaluation and decision-making process from start to finish. This group will vary by company but may involve data analysts, IT professionals responsible for product implementation and technical support, C-suite members like a Chief Data Officer, and other end users. Make sure to account for their input on what they need from a data product and how it can improve their work. As you get closer to the selection stage, jointly develop a comprehensive transition plan, with details like timelines, internal milestones, and roles and responsibilities.
And once you’re up and running with your new data product, make sure that users are given ample training and support as they transition away from the data tools they used previously. Many data product companies will offer ongoing guidance for users, giving them the tools and the resources they need to be successful when using a data product. This step is especially critical when you consider that the user-friendly nature of data products often means that a wider audience is accessing the product when compared to how many users accessed the suite of data tools. These net-new users will need to be brought up to speed on the product itself but may also need to be educated on how data products can be used in general.
By following these best practices, organizations can successfully transition from multiple data tools to a single data product, enabling them to streamline their data analysis and gain deeper insights into their data.
Data products are the future
The future of business-ready data lies in the adoption of a data product mindset for actionable data. Rather than forcing users to navigate dozens of platforms and hundreds of tables, the best data platforms unify data management and access. With a single platform for data ingestion, unifying, structuring, cleansing, validating, transforming and loading data, companies can access more comprehensive and accurate datasets, streamline their suite of tools, and simplify the experience for all users.
In addition, by considering all aspects of data utilization and ensuring that all elements are present and accounted for, companies can unlock the full potential of their data, drive better decision-making, and achieve greater success in an increasingly data-driven world.
Ready to get started with Data Products? Get in touch and we’ll make it easy!