Chapter 3: The Key Differences Between Data Lakehouses, Data Lakes and Data Warehouses

A data lakehouse emerged to serve as a single platform for the data lake and data warehousing.
Before today's advances in data management technology, businesses mainly relied on data lakes and data warehouses for business insights and storage. Though some businesses combined data warehouses with a data lake, a data lakehouse emerged to serve as a single platform for the data lake and data warehousing.
The Elements of a Data Warehouse
A data warehouse can offer some advantages to your organization. The features of a data warehouse that distinguish it from lakes and lakehouses include the following:
- Purpose: A data warehouse is ideal for business intelligence (BI) use-cases and data analytics.
- Data standardization and quality: Your company likely generates data from several sources, such as transactional data, user data and sales data. With data warehousing, you can consolidate business data into a standardized, consistent format for one source of data.
- Storage data type: A data warehouse can handle structured data.
- Business intelligence: With a data warehouse, your business can close the gap between raw data and curated data that offers business insights. These insights help you answer complicated questions about your data and make informed decisions regarding your business operations.
- ACID compliance: ACID means atomicity, consistency, isolation and durability. A data warehouse records data in an ACID-compliant way to ensure high levels of integrity.
- BI workloads and data analytics: A data warehouse increases the speed of preparing and analyzing data. The data from a data warehouse is accurate and consistent, so the warehouse can easily connect to BI and data analytics tools. A data warehouse also reduces the time needed to collect data and allows you to leverage data for analytics needs like dashboards and reports.
- Cost: A data warehouse has time-consuming, costly storage.
- Decision-making process: With data warehousing, you can improve your decision-making via a single repository of both historical and current data. You can understand your customers' needs, evaluate risks and improve your services or products with accurate insights from your data.
The Elements of a Data Lake
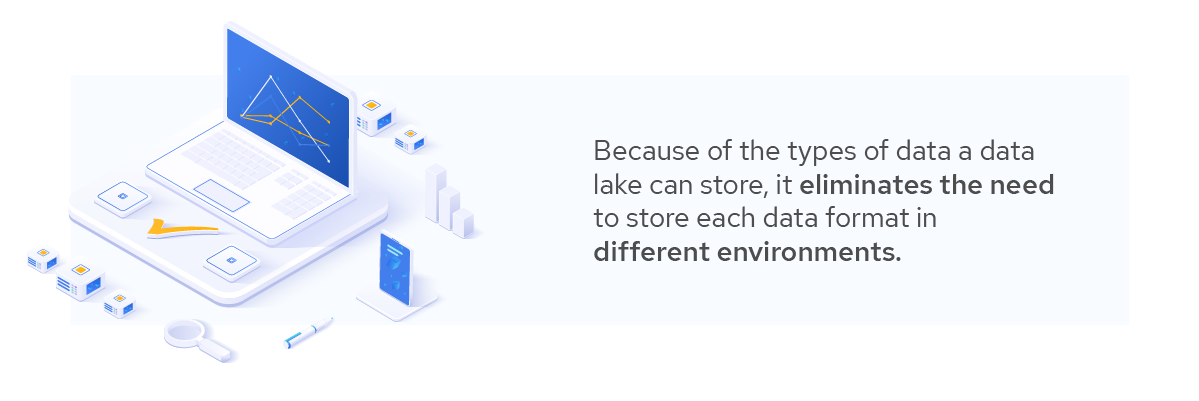
Some elements of a data lake set it apart from the other data architecture systems. The following are the features of a data lake that distinguish it from warehouses and lakehouses:
- Storage data type: A data lake can store both unstructured and semi-structured data.
- Data consolidation: Because of the types of data a data lake can store, it eliminates the need to store each data format in different environments. A data lake offers a central repository in which your business can store all types of data.
- Purpose: A data lake is optimal for artificial intelligence (AI) and machine learning (ML) workloads.
- Flexibility: A data lake is flexible, so you can store your data in any medium or format without the need for a predefined schema. When you keep your data in its original format, you'll have more data for analysis and future use.
- ACID compliance: A data lake does not offer ACID-compliance, and deletes and updates are both complex operations.
- Support for data insights: A data lake offers support for several ML and data science use cases. In a lake, data is stored in a raw, open format, which makes it easier to use algorithms to process data for meaningful insights.
- Cost: A data lake has fast, flexible and cost-effective storage. Compared to traditional data warehouses, data lakes tend to be more affordable. A data lake is designed to be stored on affordable commodity hardware.
- Inconsistency: Since a data lake lacks consistency, it can be difficult to enforce security and reliability for data.
The Elements of a Data Lakehouse
Finally, here are the features of a data lakehouse that distinguish it from data lakes and warehouses:
- Purpose: A data lakehouse is suitable for ML workloads and data analytics.
- Storage data type: A data lakehouse can handle many data types, including unstructured data, structured data and semi-structured data. With a data lakehouse, your business can reduce data duplication due to its all-purpose storage platform. This platform can cater to your business data demands and prevent the costly data duplication you might get with using both data lakes and data warehouses.
- ACID compliance: A data lakehouse is ACID-compliant and ensures consistency as several parties concurrently write or read data.
- Workload support: A data lakehouse provides support for many different workloads and offers direct access to commonly used BI tools for advanced analytics. Data lakehouses also use open-data formats with ML libraries and APIs, allowing ML engineers and data scientists to use the data.
- Cost: A data lakehouse has flexible, fast and cost-effective storage. Data lakehouses also eliminate the time and costs associated with maintaining several data storage systems.
- Data security: A data lakehouse enforces data integrity and schema, making implementing robust governance and data security mechanisms easier.

Key Differences in Data Warehouse vs. Data Lake vs. Data Lakehouse
Understanding the key differences between a data lakehouse vs. a data warehouse vs. a data lake is essential for ensuring the proper data structure in your business. The differences between a data lakehouse vs. a data lake, for example, will determine which one is better for a business than the other. Data warehouses, lakes and lakehouses can vary in their purposes, costs, storage data types and ACID compliance.
A data warehouse is the oldest storage technology for big data and has a lengthy history in reporting, analytics and BI applications. However, data warehouses struggle with unstructured data and tend to be more expensive. The data lake emerged to handle raw data in various formats on affordable storage for data science and ML workloads. Even though a data lake works well with unstructured data, it lacks the ACID transactional features of a data warehouse, making which makes ensuring data reliability and consistency difficult.
Data lakehouses are the newest in data storage architecture. Lakehouses are a combination of data lakes and data warehouses, so data lakehouses have some similarities to both. For example, data lakehouses combine the consistency and reliability of data warehouses with the flexibility and cost-efficiency of data lakes. Deciding which is the right choice for your business will depend on what types of data you have, how stakeholders and users access and utilize the data and your data sources. To test the data solutions from RightData, ask for a demo today.